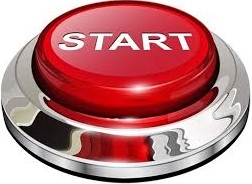
The quality of feature vector depends largely on the color space used for representation. Its property of invariance with respect to the size of the image and orientation of objects on it make it a suitable choice for feature extraction in images. Due to their little semantic meaning and its compact representation, color features tend to be more domain independent compared to other features. It is easy to extract, analyze and represent an object. It is most expressive of all the visual features. If it is used separately for each of color bands, then there will be 3*p color moments making a feature vector.įeature Vector = (Mean1, Variance1, Skewness1, Mean, Variance, Skewn, Mea, Varian ,Ĭolor is one of the most important features with the help of which humans can easily recognize images. Various feature extraction techniques haveĪll the three orders can be calculated either for each color band of image separately or for gray band. The similar features together form a feature vector to identify and classify an object. There are various types of feature extraction with respect to satellite images. This is because when a 3-D real world object is projected onto a 2-D image plane, one dimension of the object Information is lost.
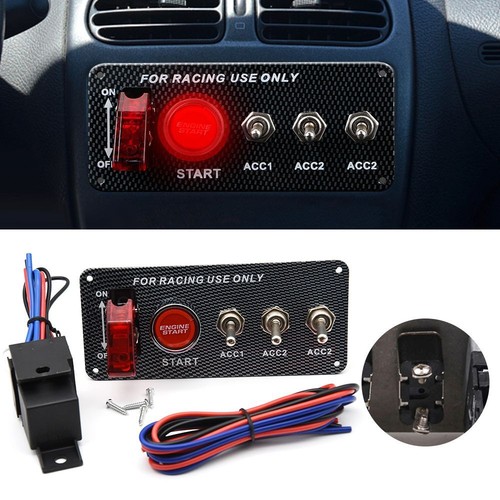
Shape representation and description is a difficult task. The first two approaches have been explored more thoroughly than shape based approaches. Due to resemblance between multi-resolution filtering techniques and human visual process, Gabor and Wavelet Transform techniques are often used for texture characterization through the analysis of spatial frequency content. Texture analysis has a long history and texture analysis algorithms range from using random field models to multiresolution filtering techniques such as the wavelet transform.

Texture features play a very important role in computer vision and pattern recognition. The texture is very important cue in region based segmentation of images. They worked with 5 partially overlapping, fuzzy regions. An approach that lies between subdividing the images and relying on fully segmented images was proposed by Stricker and Dimai. The first order (mean), the second order (variance) and the third order (skewness) color moments have been proved to be efficient in representing color distribution of images. In spite of various techniques available in literature, it is still hard to tell which feature is necessary and sufficient to result in a high performance system.Ĭolor is the first and most straightforward visual feature for indexing and retrieval of images. Feature selection is a critical issue in image analysis. Section 6 provides the conclusion.įeature extraction has a long history and a lot of feature extraction algorithms based on color, texture and shape have been proposed. Section 5 provides overview of experiments performed and results obtained using In the section 3, various FE techniques will be explained and discussed. Section 2 of the paper provides the literature survey in this area. are used to represent and index an image or an object. The various contents of an image such as color, texture, shape etc. FE is the first step to classify an image and identify the objects. FE is concerned with the extraction of various attributes of an object and thus associate that object with a feature vector that characterize it. The similarity between images can be determined through features which are represented as vector. For automatic identification of the objects from remote sensing data, they are to be associated with certain attributes which characterize them and differentiate them with each other. Mapping the image pixels into the feature space is known as feature extraction. Various techniques have been considered and their pros and cons along with the method of implementation and detailed experimental results have been discussed.įeature Extraction (FE) is an important component of every Image Classification and Object Recognition System. This paper provides a comprehensive framework of various feature extraction techniques and their use in object recognition and classification. Previous works have proposed various feature extraction techniques to find the feature vector.

This feature vector is used to recognize objects and classify them. An object is represented by a group of features in form of a feature vector. Feature Extraction for Object Recognition and Image Classificationįeature Extraction is one of the most popular research areas in the field of image analysis as it is a prime requirement in order to represent an object.
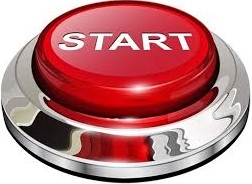